By now, most of us have integrated some form of machine learning and artificial intelligence into both our personal and professional lives. But it’s likely that these technologies are only going to become more sophisticated and impressive in the coming years.
What does the next generation of machine learning look like? And how can we prepare for it?
A Multitude of Innovators
Machine learning experts are hard to come by, in part because they’re in such great demand. Companies all over the world are looking to harness the full power of machine learning (ML) and advance it to the next level, developing the tools that are going to shape humanity for generations to come. It’s great that we have so many innovative organizations and institutions trying to push the limits of what we can accomplish. However, it’s getting harder and harder to find the ML experts you need for your own projects at a reasonable price.
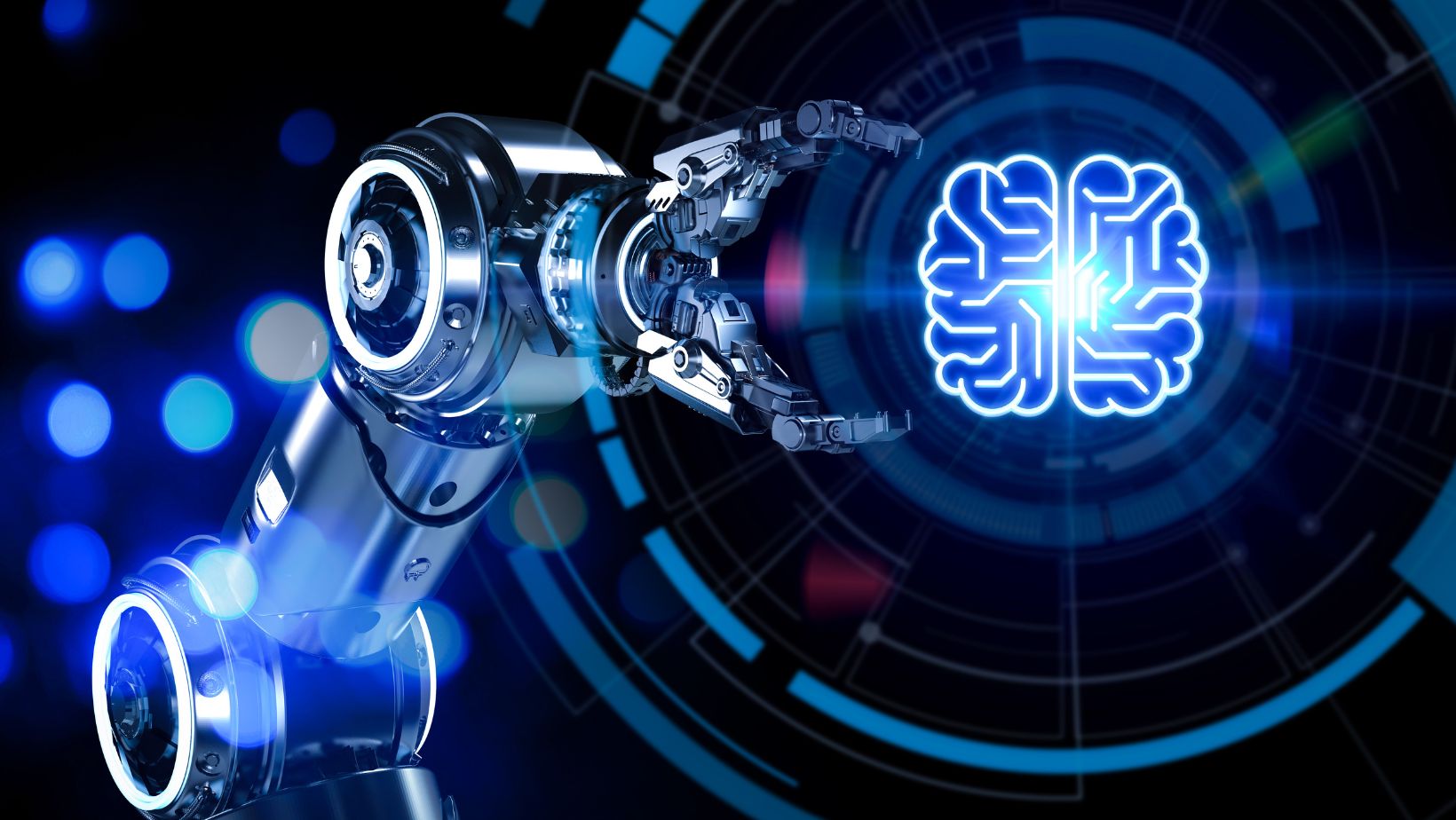
In any case, as with most technologies, it’s not exactly clear how ML is going to develop or what it’s going to look like at the end of our long road of development. All we know for sure is that ML is going to keep becoming more advanced and more sophisticated, eventually redefining how we think about work, entertainment, and more.
So what exactly could the next generation of machine learning look like?
Deeper analysis. ML technologies are analytical at their core, relying on systematic data analysis to form conclusions and eventually update themselves. Quantitative analysis at blazing speeds is a hallmark of today’s most powerful ML tools, so it’s likely that this area is going to be even more enhanced in the future. Today, if you want a deeper analysis or a faster analysis, you need to invest in computing power. But in the future, ML technology might be sophisticated enough to analyze deeper and faster while still using a similar volume of computational resources. Additionally, with a better understanding of ML and its applications, we may be able to improve the art of analysis altogether.
Flexible data requirements. Today’s ML tools require vast amounts of data for processing and analysis – at least for certain tasks. A certain engine might require thousands to millions of examples of a given concept before it truly “understands” it. But with better pattern recognition systems and increasingly complicated building block structures at the heart of ML, it’s likely that data requirements in the future will be much leaner and more flexible. It’s easy to imagine systems that can draw deeper conclusions from less data overall.
Widespread applications. Already, ML is beginning to work its way into everything. There are ML algorithms helping to decide your schedule, your search results, and even what you watch on TV at night. But in the near future, these applications are likely to become even more widespread. We may see variants of ML technology working its way into almost every corner of daily life.
Greater collaboration. With more accessible ML tools on the market, it’s likely that we’ll see a door open to greater collaboration between developers and innovators. This can lead to a more harmonious, cooperative environment for tech development.
Ethical cleanup. There are a host of ethical questions and concerns related to ML and AI. But as we become more acquainted with these technologies, including the complications and consequences of their use, it’s only natural that we’ll begin a process of ethical cleanup. Over time, we should be able to sort out many of the lingering ethical issues related to creating and using these technologies.
Novel approaches. ML and AI in the current generation aren’t fully understood – even by their own developers. However, there are some high-level approaches in programming that have come to define this technology vertical. As we anticipate future iterations of these technologies, we expect to see completely novel approaches that rebuild these ideas from the ground up. Future ML developers may conceptualize the technology in ways that would seem completely alien to us.
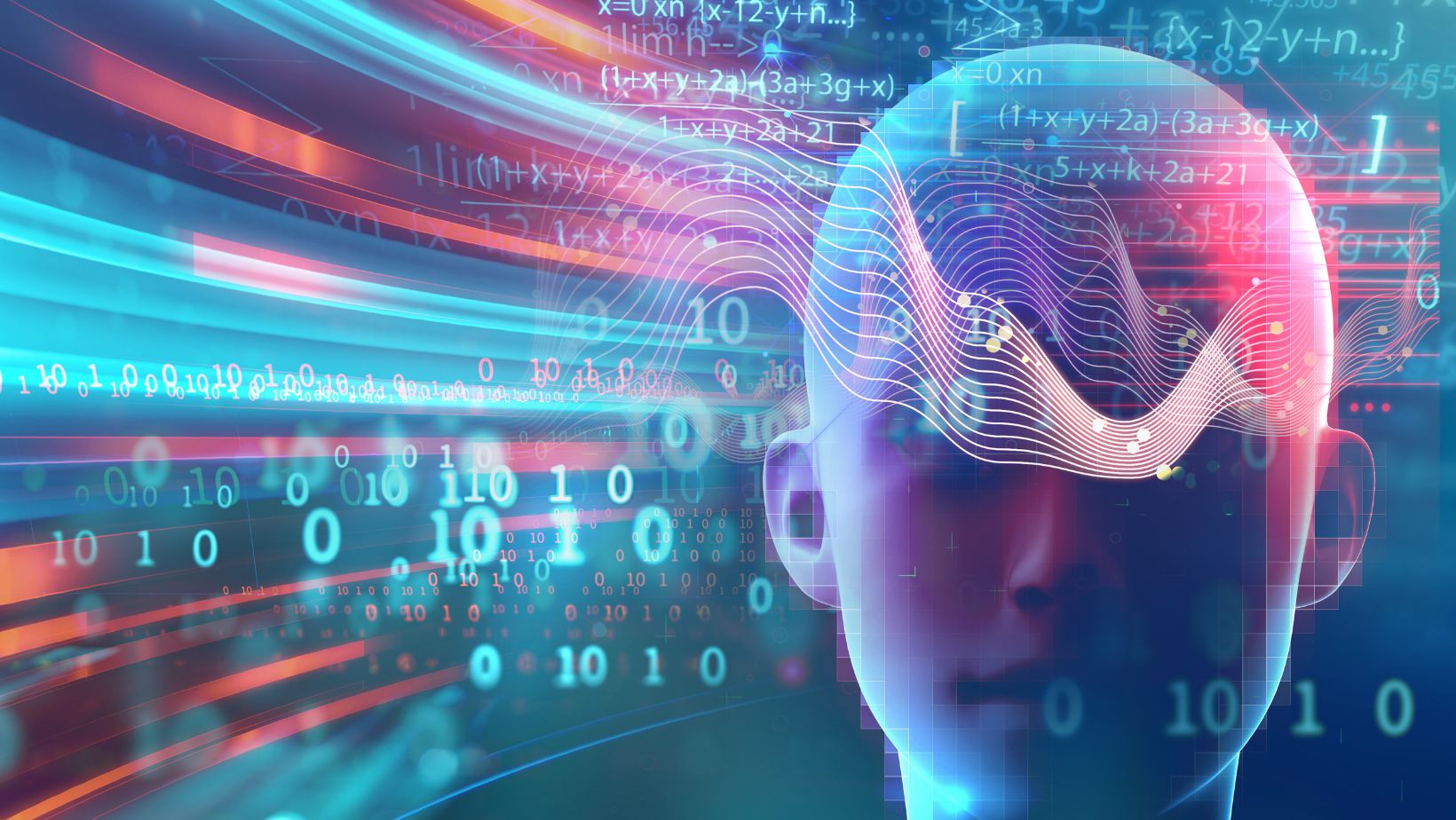
Self-development. The real taking off point is going to be when ML and AI tools can functionally improve themselves, beyond the domain limits set for them by their creators. When we have technologies ostensibly more powerful than human beings updating themselves, the rate of acceleration of technologies is going to become faster than it already is.
We don’t have a crystal ball, nor do we have enough data to sufficiently analyze the situation and make a concrete prediction about where ML goes from here. However, with some of the world’s greatest minds and top innovators on the case, it’s incredibly likely that the next generation of ML is going to dwarf this one in terms of capacity, impressiveness, and staying power.